Chatbots vs Agents: Understanding the Evolution of Conversational AI
As artificial intelligence reshapes digital experiences, two terms frequently surface in the conversation: chatbots and agents. While often used interchangeably, these two types of systems represent different stages in the evolution of intelligent interaction.
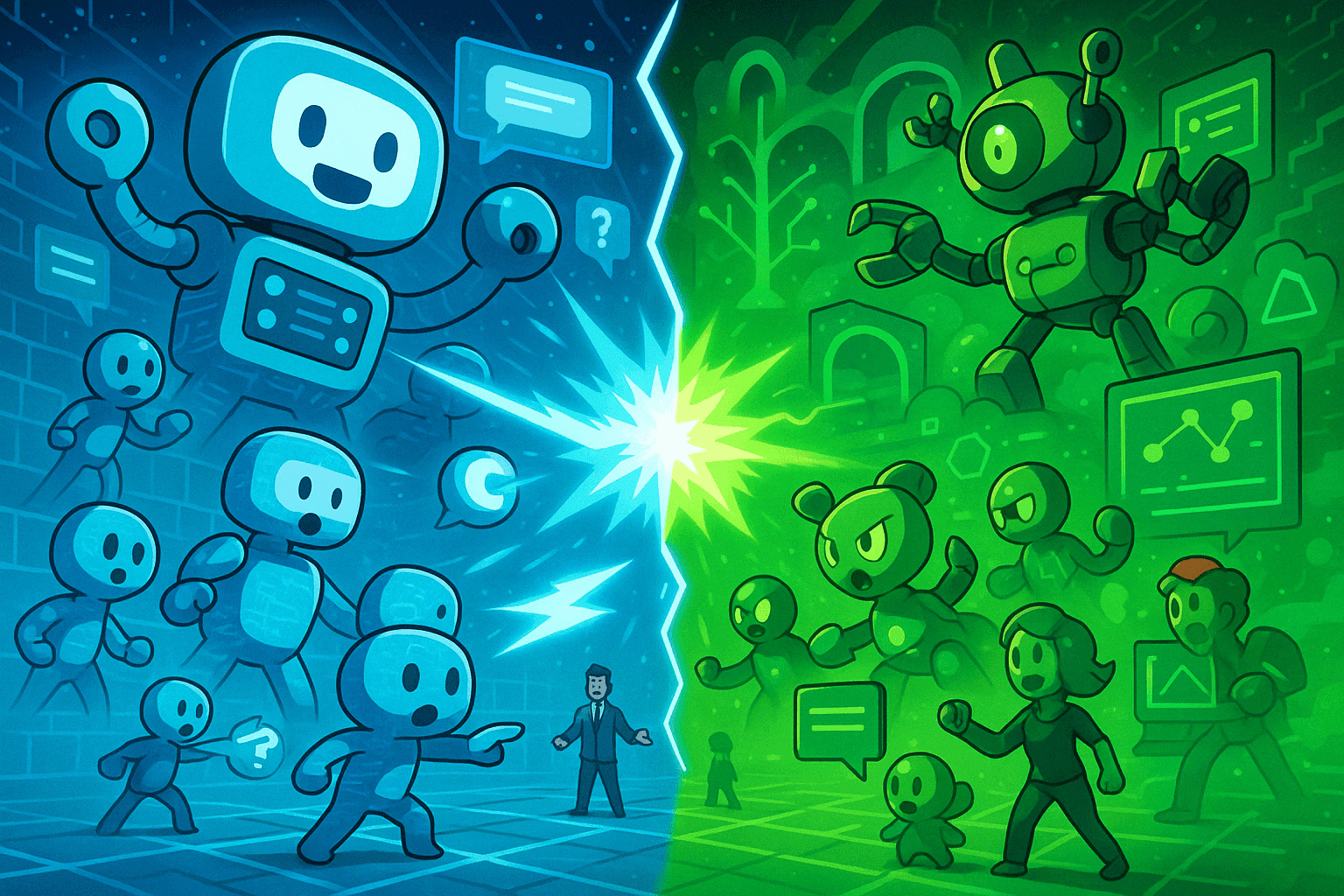
Understanding the distinction between them is key to building systems that not only respond to users—but anticipate their needs, solve complex problems, and act autonomously.
Chatbots: The First Step in Conversational AI
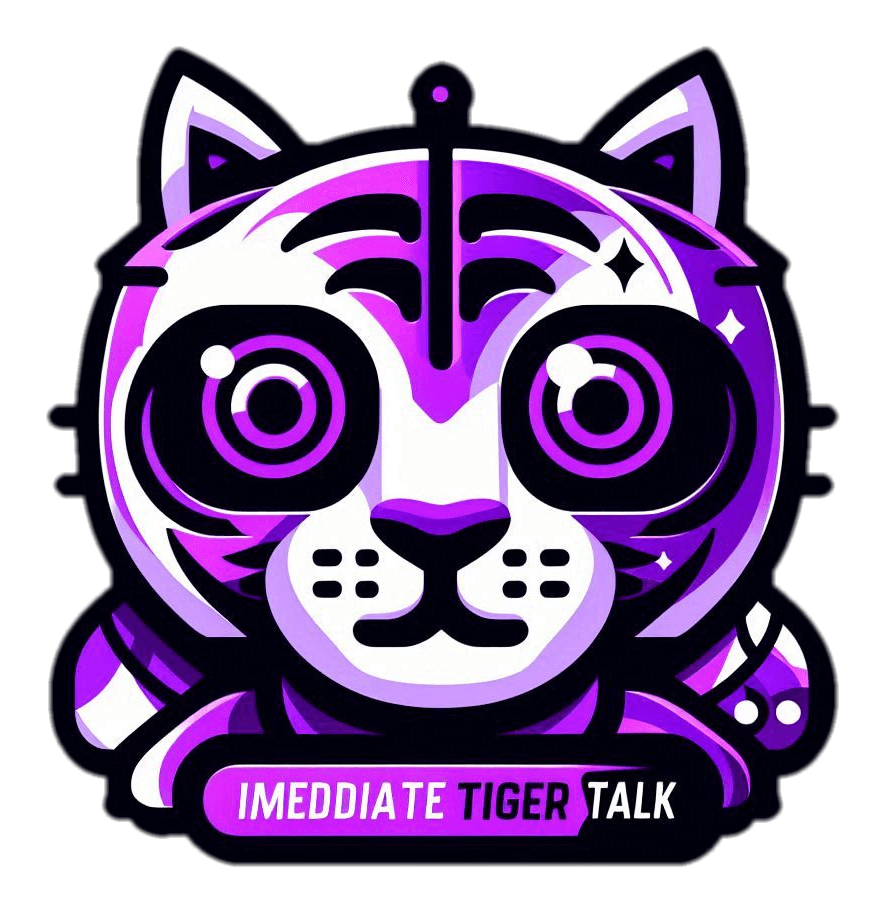
Chatbots are rule-based or AI-powered programs designed to simulate human conversation, typically through text or voice interfaces. Their main purpose is to assist users by providing quick answers, executing simple tasks, or routing queries to the right channels.
Key Characteristics of Chatbots:
Reactive: Only responds when the user initiates.
Limited Scope: Often confined to specific domains like customer support, booking systems, or product queries.
Minimal Memory: Many chatbots cannot recall past interactions or learn from user behavior.
Scripted Interactions: Responses are often predefined or trained on narrow datasets.
Examples: Website live chat support, FAQ bots, restaurant reservation bots.
Quality chatbots improve user experience with better natural language understanding (NLU) and context tracking within a session. However, they still fundamentally rely on input-response loops.
Agents: The Next Generation of Intelligent Systems
AI agents take the chatbot concept several steps further. They’re not just responsive—they’re goal-driven, autonomous, and capable of reasoning. These systems can make decisions, take proactive actions, and operate across multiple contexts or environments.
Key Characteristics of Agents:
Proactive: Can initiate actions or suggest next steps without being prompted.
Autonomous: Operates independently, pursuing goals on behalf of the user.
Multi-functional: Can use tools, access APIs, schedule tasks, or interact with other systems.
Persistent Memory: Remembers past interactions, preferences, and goals.
Examples: AI executive assistants, AI customer success reps with backend access, personal productivity agents.
Modern agents are often built using agentic AI frameworks that combine reasoning, planning, tool use, and memory into a cohesive system.
Chatbot vs Agent: A Comparison
Feature | Chatbot | AI Agent |
Initiation | User-initiated | Can self-initiate |
Scope | Narrow, predefined | Broad, dynamic |
Memory | Session-based or minimal | Persistent, long-term |
Decision-making | Reactive, rule-based | Autonomous, reasoning-enabled |
Tool use | Limited or none | Often integrated with APIs, tools, etc. |
Goal-oriented | Task-based | Outcome-driven |
Why the Difference Matters
In today’s digital landscape, users expect more than scripted responses—they want intelligent partners that understand context, reduce friction, and act in real time.
Businesses looking to scale support or automate workflows should consider the power of agents.
Developers need to understand the architectural shift from conversational interfaces to autonomous systems.
Users benefit from richer, more personal, and more helpful experiences.
Conclusion
The journey from chatbot to agent mirrors the evolution of AI itself—from static, rule-based systems to intelligent, dynamic actors. While chatbots are still useful in many applications, agents represent the future: adaptive, autonomous, and deeply integrated into our digital lives.
As you design your next AI system, ask yourself not just, “Can it chat?” but “Can it act?”
Personally I will stick only to chatbots for now.